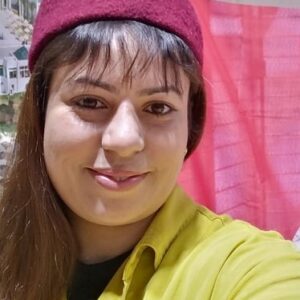
Contact
Courriel : asma.dhaouadi@univ-smb.fr
Téléphone : +33(0) 765228805
Bureau : A221-A222
Adresse 1 : LISTIC – Polytech Annecy-Chambery, BP 80439, 74944 Annecy le Vieux Cedex, France
Thèse
Thème : Modélisation du Data Warehousing dans le contexte du Big Data
Résumé :
Dans l’ère du Big Data, la rapide évolution des technologies rend le choix des outils adapté aux besoins des experts complexe. Les études existantes sur le stockage et l’analyse de données Big Data sont souvent limitées et spécifiques. Pour surmonter ces limites, cette thèse propose une approche de modélisation générique, inspirée de l’architecture dirigée par les modèles, pour guider le choix technologique à chaque étape. Un cadre interactif aide à modéliser un pipeline personnalisé et à vérifier l’interopérabilité des outils, avec recommandations de connecteurs. Ce cadre a été validé à travers des études de cas sur le COVID-19 et la Coupe du Monde 2022. Les retours des utilisateurs sont satisfaisants (85 % de satisfaction).
ABSTRACT :
Mots clés : Data Warehouse, ETL Process Modeling, Data Warehousing Architectures, Knowledge Discovery, Meta-Model, Generic Methodology
Dhaouadi, A., Bousselmi, K., Monnet, S., Gammoudi, M. M., & Hammoudi, S. (2024). A Machine Learning Based Decision Support Framework for Big Data Pipeline Modeling and Design. The Jordanian Journal of Computers and Information Technology (JJCIT), Vol. 10, No. 03, pp. 306 – 318. — https://www.jjcit.org/paper/232/A-MACHINE-LEARNING-BASED-DECISION-SUPPORT-FRAMEWORK-FOR-BIG-DATA-PIPELINE-MODELING-AND-DESIGN
Dhaouadi, A., Paccoud W., Bousselmi, K., Monnet, S., Gammoudi, M. M. & Hammoudi, S. (2023). Big Data Tools: Interoperability Study and Performance Testing. IEEE Big Data 2023.– https://ieeexplore.ieee.org/document/10386089>
Dhaouadi, A., Bousselmi, K., Gammoudi, M. M., Monnet, S., & Hammoudi, S. (2022). Data Warehousing Process Modeling from Classical Approaches to New Trends: Main Features and Comparisons. Data, 7(8), 113. — https://doi.org/10.3390/data7080113
Dhaouadi, A., Bousselmi, K., Monnet, S., Gammoudi, M. M., & Hammoudi, S. (2022). A Multi-layer Modeling for the Generation of New Architectures for Big Data Warehousing. In International Conference on Advanced Information Networking and Applications (pp. 204-218). Springer, Cham. https://doi.org/10.1007/978-3-030-99587-4_18
Dhaouadi, A., Gammoudi, M. M., & Hammoudi, S. (2019). A Two Level Architecture for Data Warehousing and OLAP Over Big Data. Proceedings of the 34th International Business Information Management Association (IBIMA), ISBN: 978-0-9998551-3-3, 13-14 November 2019, Madrid, Spain, p. 7182-7194. https://ibima.org/accepted-paper/a-two-level-architecture-for-data-warehousing-and-olap-over-big-data/
Encadrant : Sébastien Monnet & Mohamed Mohsen Gammoudi
Co-encadrant : Khadija Arfaoui
Début de la thèse : Janvier 2021